Machine Learning, AI and Risk Management: TIR34971 Explained
TIR34971:2023, Application of ISO 14971 to machine learning in artificial intelligence, was published in March 2023.
The technical report is a response to the AI boom, which has added a layer of complexity to complying with regulations and standards in the MedTech industry that don’t specifically cover newer technologies like AI.
And as machine learning (ML) becomes more prevalent in diagnostics and treatment recommendations, the MedTech industry needs guidance on how to stay aligned with standards like ISO 14971 while designing and developing devices that use ML.
That’s where TIR34971:2023 comes in. This report aims to provide medical device manufacturers with guidance on how they can follow the risk management process in ISO 14971 while building devices that use machine learning.
What is TIR34971 and who should use it?
TIR34971 is a technical report that is intended to serve as a companion to ISO 14971:2019. The technical report does not provide a new risk management process or expand the requirements laid out in ISO 14971:2019.
It does, however, provide guidance for manufacturers who need to understand how they can apply ISO 14971 to regulated AI medical technologies. In other words, if your device uses machine learning, this guidance will help you consider the unique risks associated with the ML component of your device and how you should go about evaluating and controlling those risks.
What are some key considerations in the AI risk management process?
The structure of TIR34971 follows the risk management process outlined in ISO 14971. Again, there’s nothing new here in terms of the requirements listed in ISO 14971:2019. As the technical report notes, “Classical risk management approaches are applicable to ML systems.”
That said, using machine learning in a product creates a large amount of potentially new and unfamiliar risks for end users.
For instance, risk analysis still starts with identifying and documenting a clear description of your device and its intended use. But if your device is using machine learning, the questions you ask about intended use and foreseeable misuse are a little different than they would be for a traditional medical device.
TIR34971 notes that you may need to ask questions, like:
-
Is this software providing diagnosis or treatment recommendations? How significant is this information in influencing the user? Does it inform or drive clinical management?
-
Does the system offer a non-ML implementation of the same functionality?
-
Does this device have any autonomous functions? What is the level of autonomy?
-
Does this device have the ability to learn over time? Can it adjust its performance characteristics, and if so, what are the limits on that adjustment?
-
If the system is intended to learn over time, will that potentially affect its intended use?
Section 5.3 of the report, Identification of characteristics related to safety, contains a wealth of information on what to watch out for when working with AI and machine learning.
Data management
Since medical devices based on machine learning need to be “trained” on data before their use with patients, there are a number of risks associated with data management. This is a lengthy section, but a few of the most critical issues with data management include:
-
Incorrect data
-
Incomplete data
-
Incorrect handling of outliers in the data
-
Inconsistent data that varies from source to source, for instance
-
Subjective data that has been influenced by personal beliefs or opinions
-
Atypical data that does not represent the quality of data in the device’s actual use
Bias
Bias is a major source of potential risk when it comes to the performance of a medical device that uses machine learning. While not all bias is inherently negative, there are a number of different ways that the data used to train ML algorithms can be biased in such a way as to affect their safety and effectiveness.
These biases include:
-
Implicit bias
-
Selection bias
-
Non-normality
-
Proxy variables
-
Experimenter’s bias
-
Group attribution bias
-
Confounding variables
Data storage, security, and privacy
This refers to the fact that the data used during the development, testing, and training of an ML application may be an attractive target for malicious actors.
And as TIR34971 notes, “While many of the techniques for assuring cybersecurity will apply to ML, due to the opaque nature of ML, it can be difficult to detect when security has been compromised.” This means any MedTech company using ML in their devices will need to monitor their products’ performance and take appropriate measures to ensure privacy, such as the use of synthetic data.
Overtrust
Overtrust refers to the tendency of users to develop confidence in technology to the point that they become overly dependent on it and trust the technology even when circumstances indicate they should be skeptical.
Overtrust can happen when users:
-
Develop too much confidence in the application’s ability.
-
Perceive risks to be lower than they are and delegate to the application.
-
Have a heavy workload. You’re more likely to trust the application when you don’t feel you have time to do something yourself or double-check its work.
-
Have low confidence in their own ability and defer to the application.
Adaptive systems
An adaptive system that learns over time as it continues to collect data creates new challenges regarding change control for the medical device. TIR34971 suggests that manufacturers should adopt methods that support continuous validation of these adaptive algorithms, potentially allowing them to roll back to prior validated models if performance falls outside accepted metrics.
Remember, while these characteristics related to safety may be unique to devices using machine learning, the risk management process outlined in ISO 14971 has not changed.
TIR34971 also includes information on applying what you learn in your risk analysis to your risk evaluation, risk controls, evaluation of residual risk, risk management review, and production and post-production activities.
What’s included in TIR34971 annexes?
As is often the case, the annexes in TIR34971 provide clarification of concepts and examples of what’s been discussed in the main body of the text.
-
Annex A is simply an overview of the risk management process according to ISO 14971, which may seem basic, but is great to have as a reference while you’re working your way through the technical report.
-
Annex B provides examples of machine-learning related hazards, as well as the relationships between those hazards, their foreseeable sequence of events, hazardous situations, harms, and risk control measures.
-
Annex C specifies some of the personnel qualifications that might be required to appropriately carry out the risk management process with a device that has an ML component.
-
Annex D covers the considerations for autonomous systems. This is an important element of your risk management because the level of autonomy in a system affects the risks of the device. Medical devices with a degree of autonomy will need specific risk control measures to ensure their safety and effectiveness.
Choose an AI-powered risk management solution designed exclusively for medical devices
At Greenlight Guru, we know that risk management is more than just another checkbox activity; it’s an integral part of the entire medical device lifecycle.
That’s why we’ve built a first-of-its-kind tool for risk management—made specifically for MedTech companies. Greenlight Guru’s Risk Solutions provides a smarter way for MedTech teams to manage risk for their device(s) and their businesses.
Aligned with both ISO 14971:2019 and the risk-based requirements of ISO 13485:2016, our complete solution pairs AI-generated insights with intuitive, purpose-built risk management workflows for streamlined compliance and reduced risk throughout the entire device lifecycle.
Ready to see how Risk Solutions can transform the way your business approaches risk management? Then get your free demo of Greenlight Guru today.
Etienne Nichols is the Head of Industry Insights & Education at Greenlight Guru. As a Mechanical Engineer and Medical Device Guru, he specializes in simplifying complex ideas, teaching system integration, and connecting industry leaders. While hosting the Global Medical Device Podcast, Etienne has led over 200...
Related Posts
What are the Risk Management Documentation Requirements of ISO 14971?
Can dFMEA and ISO 14971 Co-Exist in Medical Device Risk Management?
Understanding ISO 14971 Medical Device Risk Management
Get your free download
ISO 14971:2019 Gap Analysis Tool
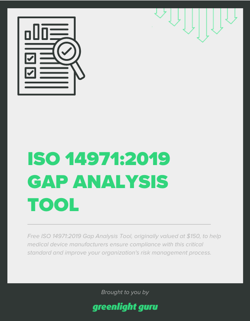