Most of us are quite comfortable using automations in both our personal and work lives. So much so that we barely think of them as automations anymore.
When you set a reminder on your phone to change the air filter in your furnace every three months, you’re using automation. When you complete a task in your project management software, and it sends a message to a colleague that you’ve done so, that's automation as well.
The interesting thing is that while we’re comfortable with automation, there’s a lot of skepticism around the many AI tools that have come out recently. For example, Greenlight Guru recently surveyed more than 200 MedTech professionals about their use of AI tools and their attitudes toward them. And while the vast majority were interested in these tools, less than a third of our respondents were actually using them. Many expressed hesitancy about their accuracy and the potential for errors.
Skepticism of AI is understandable, but some of it appears to rest on a misconception about what it is these AI tools are actually doing. There’s a misunderstanding regarding the relationship between automation (which we all accept and often love) and AI (which we are generally wary of).
I think if we consider the close relationship between the two, as well as how AI differs from simple automations, we can begin to ease some of that wariness and help people feel more comfortable with the vast potential of these tools.
Automation vs. AI: A Continuum, Not a Divide
At its core, automation means executing a predetermined action in response to a specific trigger. You can think of it as a simple cause-and-effect mechanism; if you perform a particular action, the software will respond in a predefined manner. This deterministic model has been the backbone of our software interfaces for ages.
AI is often thought of as an advanced system that can think, learn, and make decisions independently. However, in reality, present-day AI is really more of a continuation of automation, with the primary distinction being complexity of the inputs and outputs.
Consider the process of interpreting text. Traditional automation might involve reacting to a simple input like a 'yes' or 'no' response, but AI can evaluate an entire paragraph to discern its intent and decide the best course of action based on this complex non-fixed input. And when there is a high degree of non-determinism—meaning the input is less structured or more random—AI can still effectively process it and determine the appropriate response.
Think about your experience calling an automated customer service line. If you’re prompted to speak at all, it’s typically in one or two word answers, like 'yes' or 'no' that will eventually lead to a recorded response or perhaps a customer service agent. That automated system can’t handle much non-determinism; you can’t patiently explain your problem to it and expect it to provide an answer.
In MedTech, an automation in a medical device might assess a simple blood measure, but you would need an AI system to evaluate brainwave activity or analyze radiology data and then offer diagnostics or insights from the data.
In other words, once you reach a certain level of complexity, leveraging AI to handle the analysis becomes the most efficient solution because writing a deterministic software program to accomplish the task becomes intractably complex because of the number of steps and branching logic involved.
Understanding the risks is key to using (or not using) AI
For those comfortable with software automations but wary of AI, it's worth noting that the risks associated with AI are not always greater than traditional systems. It boils down to the potential cost of an algorithm making the wrong decision.
Many AI systems have been proven to have error rates lower than humans in a litany of tasks. However, people's perceptions of risk, especially in unfamiliar areas, can be skewed by lack of data and personal experiences. There are a couple cognitive biases that come into play here and are worth examining.
Availability bias
The first is what’s known as availability bias, which is simply the tendency of people to deem events they can easily recall as more probable. For instance, if you’ve recently seen several news articles about self-driving cars crashing or otherwise malfunctioning, you’re more likely to consider them highly dangerous. Or if you’ve used ChatGPT a handful of times and seen it “hallucinate” an answer, that experience may quickly come to mind when you’re evaluating the risk of another AI tool.
Anchoring bias
The second is anchoring bias, which occurs when people become fixated on an initial piece of information and disregard updated data that conflicts with their initial assessment. A poor first experience can easily become the anchor that determines whether we think an AI tool, like a large language model (LLM), for instance, can be of use to us—regardless of its true potential.
The net effect is that these types of biases will cause folks to vastly overestimate the risks of using these systems in relation to the actual probability and likely severity of harm. Overcoming these biases isn’t always easy, but recognizing them is necessary if we want to get a real understanding of the true risks and benefits of any AI application.
As with any complex system, a benefit-risk analysis needs to be performed before people begin using it. And there are ways to statistically evaluate the risks of AI, either in producing medical devices or in a medical device itself. Once we understand that benefit-risk trade off, we can make informed decisions about the use of AI (or any automation for that matter) in a device.
How should medical device companies assess and control the risks of AI?
To help us understand the actual risks involved in the use of AI in medical devices—and how MedTech companies are working to mitigate those risks—I asked Wade Schroeder, Manager of Guru Services here at Greenlight Guru, to tell us a little more about how MedTech companies should think about these risks.
Wade pointed out that the major safety risk related to the use of AI in medical devices is using “unbounded” AI (in which there are no predetermined boundaries for modifications due to machine learning). That’s why the use of a Predetermined Change Control Plan (PCCP), as outlined in recent FDA guidance, is so important.
“MedTech companies should create pre-specifications to draw a region of potential change and an Algorithm Change Protocol,” Schroeder said. “Those two documents should include information about Data Management, Re-Training (machine learning methods), performance evaluations and procedures for updates.”
In its preface to the PCCP guidance, FDA states that it continues to receive an increasing amount of submissions for AI/ML-enabled medical devices. It’s clear that we are going to see more devices hitting the market that will help with decision-making for doctors and other medical professionals, assisting in making more accurate diagnosis and treatment of diseases. But Schroeder also sees the potential for AI to move from its current siloed use cases into helping with more holistic medical plans to help patients proactively test and manage their health.
As we continue to move closer to that future, and begin seeing more and more AI-enabled medical devices on the market, it’s important to keep in mind that this technology isn’t something we’ve never seen before. Rather, it’s an extension of the automated processes we’ve all grown accustomed to.
By approaching AI with an open mind, we can objectively consider both its risks and potential benefits and make appropriate decisions about where to use it and how to mitigate its risks.
AI-powered risk management solution purpose-built for medical devices
At Greenlight Guru, we know that risk management is more than just another checkbox activity; it’s an integral part of the entire medical device lifecycle.
That’s why we’ve built a first-of-its-kind tool for risk management—made specifically for MedTech companies. Greenlight Guru’s Risk Solutions provides a smarter way for MedTech teams to manage risk for their device(s) and their businesses.
Aligned with both ISO 14971:2019 and the risk-based requirements of ISO 13485:2016, our complete solution pairs AI-generated insights with intuitive, purpose-built risk management workflows for streamlined compliance and reduced risk throughout the entire device lifecycle.
Ready to see how Risk Solutions can transform the way your business approaches risk management? Then get your free demo of Greenlight Guru today.
Tyler Foxworthy is the Chief Scientist at Greenlight Guru, where he applies his expertise in AI and data science to develop new MedTech products and solutions. As an entrepreneur and investor, he has been involved in both early and late-stage companies, contributing significantly to their technology development and...
Related Posts
Guide to Understanding AI Applications that Drive Business Value in MedTech
What is a Predetermined Change Control Plan (PCCP) and Who Needs One?
2025 Medical Device Industry Report: Quality Challenges, Regulatory Complexity, and Economic Uncertainty
Get your free PDF download
ChatGPT Sample Prompts for Risk Evaluation of a Medical Device
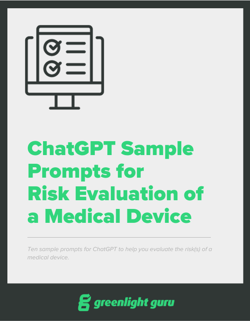